We proposed a stock vector representation called “stock embedding,” obtained using a deep learning framework that utilizes news articles and stock price history. This embedding is applicable to financial problems beyond price prediction. As an example, portfolio optimization using Reuters and Bloomberg headlines resulted in a capital gain 2.8 times greater than that achieved using traditional methods with only stock price data. This result suggests that the proposed stock embedding can leverage textual financial semantics to solve financial prediction problems.
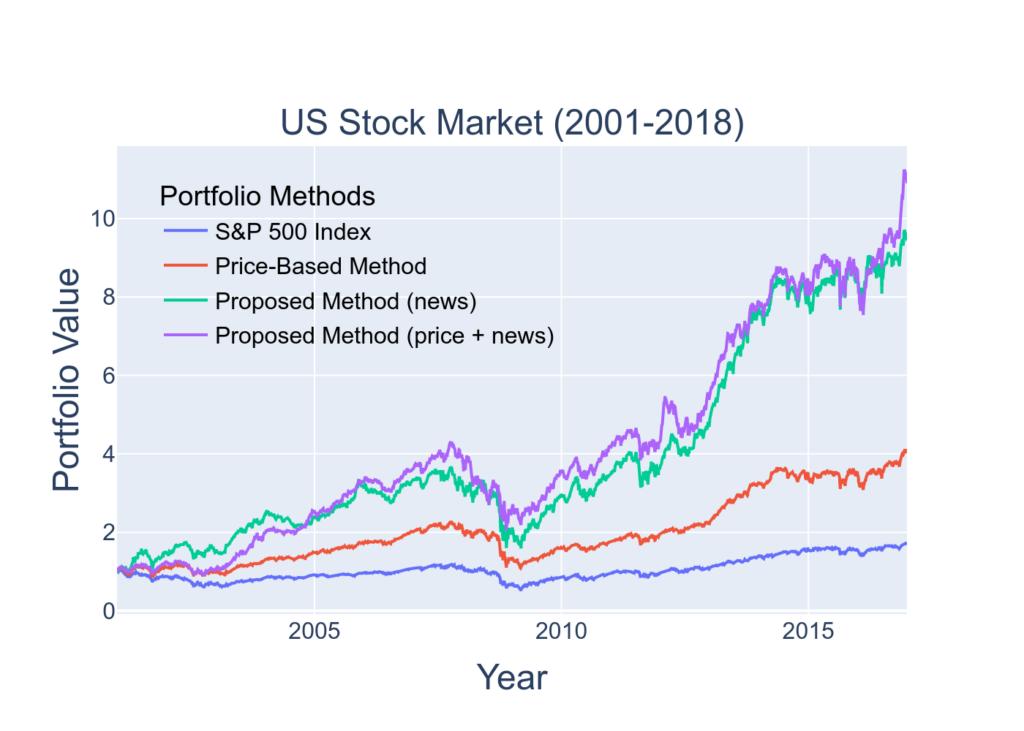
References
- Xin Du and Kumiko Tanaka-Ishii. Stock embeddings acquired from news articles and price history, and an application to portfolio optimization. In Proceedings of the 50th Annual Meeting of the Association for Computational Linguistics (ACL) Long Papers, 2020. p. 3353-3363. [link]