We explore the complexity underlying human symbolic sequences via entropy rate estimation. Consider the number of possibilities for a time series of length n, with a parameter h, as 2_hn_. For a random binary series consisting of half ones and half zeros, h=_1. For the 26 characters in English, however, the number of possibilities is not 26_n, because of various constraints such as “q” being followed only by “u”. Shannon computed a value of h=1.3, but the question of acquiring a true h for human language is difficult to answer and remains unsolved: in fact, it is unknown whether h is even positive. Therefore, we study ways to compute the upper bound of h for various kinds of data, including music, programs, and market data, in addition to natural language.
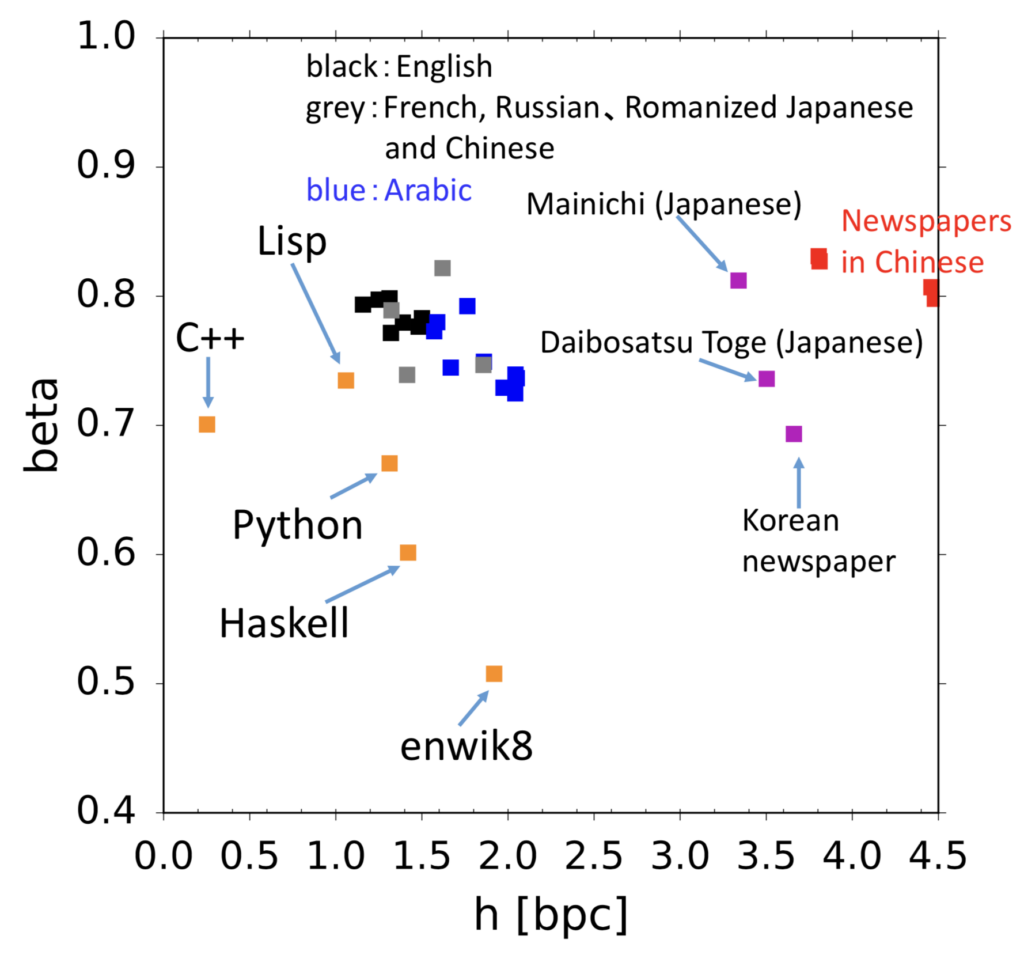
References
- Ryosuke Takahira, Kumiko Tanaka-Ishii, and Łukasz Dębowski. Entropy Rate Estimates for Natural Language—A New Extrapolation of Compressed Large-Scale Corpora. Entropy, 2016, 18.10: 364. [link]
- Geng Ren, Shuntaro Takahashi, Kumiko Tanaka-Ishii. Entropy Rate Estimation for English via a Large Cognitive Experiment Using Mechanical Turk. Entropy, 2019, 21.12: 1201. [link]